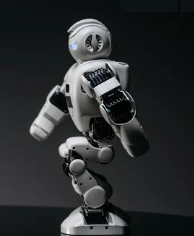
Fetch.ai has just presented the ASI-1 Mini product, which is a large language model with Web3 integration developed to agentic workflows and artificial intelligence through automation and decentralized intelligence enhancement. This singular is a crucial necessity for contemporary manufacturers. The returns are in line with the best top variants, and at the same time, the functioning is possible without an enormous amount of money investment. By means of the cost reduction of the hardware, ASI-1 Mini increases the implementation of AI solutions in the enterprise, which is commonly considered high-performance with the scalable and cost-effective ones.
Seamless Web3 Integration for Autonomous AI Operations
A completely new release of ASI-1 Mini—an AI system that is able to work in Web3 ecosystems—is being introduced. The newly unveiled Cortex suite constantly extends and upgrades AI and general intelligence functionalities.
Democratizing AI Ownership via Web3
A key highlight for Fetch.ai is its core concept of decentralizing AI model ownership. It lets the Web3 community train, control, and profit from foundational AI models. With the AI model, a person can directly contribute to the growth of AI technology and obtain a premium for his/her participation while the ASI-1 Mini and the other models like it scale to multi-billion-dollar valuations. Fetch.ai is a platform that also allows users to easily invest in and train and monetize the AIs, and this is how AI is built and owned.
Adaptive Reasoning & Optimized Task Execution
ASI-1 Mini has four dynamic reasoning modes, which involve it being flexible enough to change its approach to solving problems depending on the task complexity:
- Multi-Step Reasoning requires the system to be designed in a way that it can carry out operational steps until the problem is resolved.
- Complete Reasoning leads to clear and succinct responses to a given situation.
- Optimized Reasoning is the process of managing data to come up with a suitable inference using as few computational resources as possible.
- Short Reasoning aims to come up with to-the-point considerations that are finally translated into concrete actions.
Apart from the above, the model can be hierarchical as it allows for the incorporation of two other plants:
- Mixture of Models (MoM): It accomplishes task input feature filters and weights of the newest selected by the user to optimize running cluster and servers of AI exercising multi-modal and federated learning.
- Mixture of Agents (MoA): It is a combination of individual AI agents with unique reasoning capacities. Just as people need to work in teams for complex tasks, the agents finally need to integrate their capacities to undertake intellectual tasks.
Scalability & Cost-Effective Performance
Contrary to very computerized Language Learning Models, ASI-1 Mini is designed to save energy when it works on only two GPUs due to its highly efficient design. This is a total reduction of eightfold hardware requirements. The benchmarking has rolls topping results that clearly show that the achievements of ASI-1 Mini are on par with the leading AI models not only in the medical field but also in the history, business, and logical reasoning sectors.
The future upgrades of the model will bolster its context window to a level that will be able to support more extensive datasets as the context window grows:
- 1 Million Tokens: Used for the purpose of annotating intricate scientific documents and technical specifications, e.g., analyzing the documentation necessary to scale AI models and run AI experiments.
- 10 Million Tokens: Large enterprise applications integrating AI, particularly in the case of legal document reviews, financial data processing, and analysis, etc., will be able to run with efficiency and speed.
Enhancing AI Explainability & Transparency
There has never been enough clarity in AI due to the appearing problem of the “black box,” which emits obscure responses from AI models without showing the underlying rationale. ASI-1 Mini solves this problem through the application of continuous multi-step reasoning, which provides a clear way of reasoning—writing to relevant domains such as finance and healthcare.
The multi-expert model design of the ASI-1 Mini also improves business automation through the optimization of smooth workflow automation, thus reducing the risks of failure and enhancing decision-making speed through real-time data processing, business logic executions, and autonomous system management.
AgentVerse: Building an AI-Driven Autonomous Economy
Drifting towards the integration of ASI-1 Mini with Fetch.ai’s decentralized AI marketplace, AgentVerse gives users the opportunity to deploy micro-agents, AI-driven on the server, that can carry out real-life tasks through natural language commands.
This whole ecosystem envisions and puts into practice an AI-powered digital economy that benefits software developers and allows businesses to use automation in situations such as information processing, payments, travel, and transaction planning, and decision-making based on data.
In parallel with the ongoing improvements, ASI-1 Mini aims to become a leading multitask AI application that can learn and process structured data, text, images, and complex datasets. At the same time, it will be able to make context-aware autonomous decisions for the next generation of AI applications.